26 февраля 2023
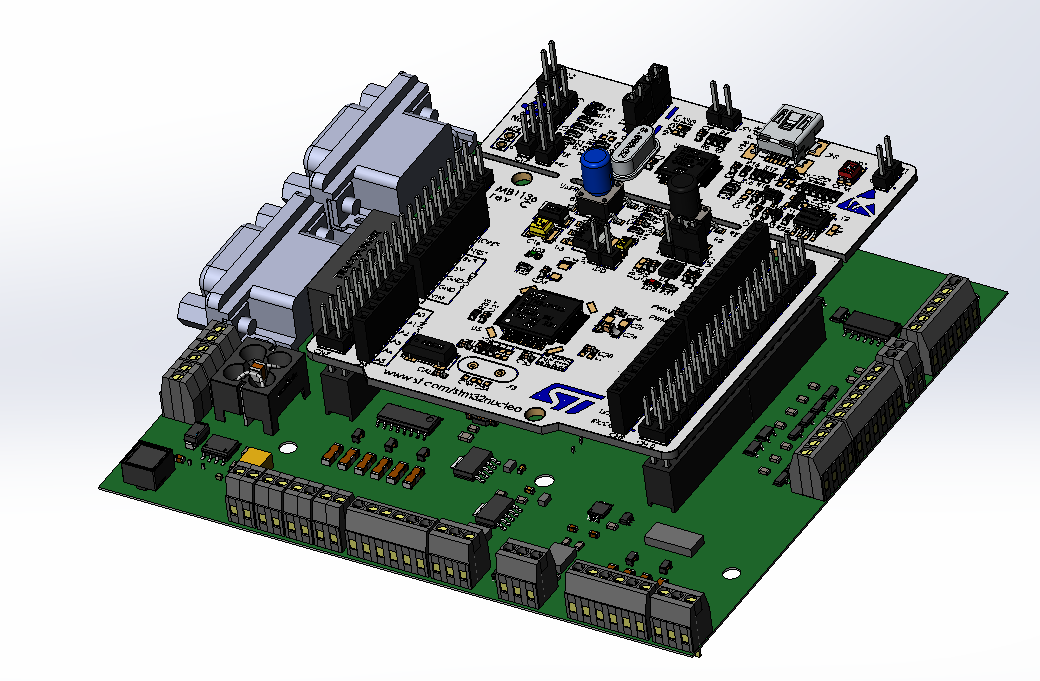
Лаборатория киберфизических систем Университета ИТМО является быстрорастущим и развивающимся
подразделением, объединившим профессионалов в области робототехники, программирования,
безопасности информационных технологий.
Стратегической целью лаборатории является выход на международный научный уровень и решение
сложных прикладных задач в области разработки и исследования киберфизических систем различного назначения.
26 февраля 2023
5 мая 2024
8 апреля 2024
A linear time-varying state observer is presented for a chain of integrators having bounded disturbances in the last equation. It is demonstrated that in the noise-free setting, for the continuous-time realization, the estimation error converges to zero with a hyperexponential rate (faster than any exponential) uniformly in the disturbance. An implicit discretization scheme of the observer is proposed, which in the discrete time preserves all main properties of the continuoustime counterpart. In addition, the discrete-time estimation error is robustly stable with respect to the measurement noise. The efficiency of the suggested observer is illustrated through comparison with a linear high-gain observer and a sliding mode high-order differentiator.
This paper investigates ML methods for diagnosing failures in an integrated Inertial Navigation System (INS) of the underwater vehicle ODIN UAV. Using dynamic equations of motion and considering measurement noise, a dataset was generated during normal operation and during various failures. Data preprocessing, exploratory analysis and feature engineering were conducted. To solve the problem of INS technical state classification, multiple machine learning models were evaluated and optimized using training data and techniques to reduce the number of dimensions. A comparative analysis of the obtained results was carried out. The paper contributes insights into effective ML-based diagnostics for enhancing navigation system reliability in autonomous underwater robots.
Self-driving vehicles are capturing the attention of numerous researchers, companies, and car enthusiasts. The automation of vehicles varies across multiple levels, depending on the tasks they perform and the techniques they employ. The Adaptive Cruise Control (ACC) system is an automated vehicle at the first level. It checks the presence of other vehicles in its lane to adapt its velocity accordingly or maintain a constant speed. However, it does not include lane-changing capabilities. Nevertheless, the presence of other neighboring vehicles can affect the performance of the ACC based Model Predictive Controller (MPC) system, leading to discomfort or collisions. In this paper, we will provide a brief description of the ACC system and the MPC controller. Subsequently, we will discuss the challenges that affect the performance of the ACC based MPC system and propose potential solutions using the Variable Time Headway (VTH) strategy or changing-lane prediction algorithms, such as Random Forest (RF), K- Nearest Neighbor (KNN) and Support Vector Machine (SVM). Simulation results of applying these methods to a sufficiently dangerous driving scenario will be illustrated, followed by a brief conclusion.